top of page
Student Researcher at Google LLC and Earth System Science Ph.D. Candidate at UC Irvine
CV | Google Scholar | ResearchGate | Github
Simple Neural Network Emulation of Convection under Full Complexity Real Geography
ML Replacement of Sub-Grid Convection: Text
Deep Neural Networks have shown great promise in learning to replace sub-grid parameterizations of convective tendencies on simple aqua-planets. Can these simple machine learning models work under more realistic, earth-like conditions?
ML Replacement of Sub-Grid Convection: Research

ML Replacement of Sub-Grid Convection: Image
Leveraging formal, automated hyperparameter tuning, Sherpa (and sufficient training data), we find even simple neural networks can capture the major variations behind heating and moistening tendencies locally in space and time. In particular, our simple neural networks are very skillful on the diurnal to synoptic timescales; largely agnostic to modes.
ML Replacement of Sub-Grid Convection: Research
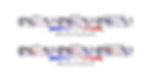
ML Replacement of Sub-Grid Convection: Research
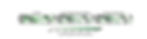
ML Replacement of Sub-Grid Convection: Video
Unfortunately, as the animations above indicate, while the neural network is skillful at capturing dominant variations in convective behavior, stochastic patterns are much more challenging. Additionally, our tests show the importance of considering the physics of the variables we are asking the neural network to emulate. For example, precipitation is a positive definite variable but the neural network does not know that unless we enforce it either in the network architecture or loss function
ML Replacement of Sub-Grid Convection: Research
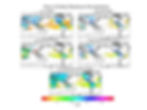
ML Replacement of Sub-Grid Convection: Image
But despite imperfections in emulating the fastest variations of convection, the overall strong performance of our DNN indicates it may be an ideal candidate for coupling to potentially power a climate model in running online simulations.
ML Replacement of Sub-Grid Convection: Research
Collaborators
ML Replacement of Sub-Grid Convection: Team Members
ML Replacement of Sub-Grid Convection: Pro Gallery
bottom of page